Through greater investment, formation of partnerships, and continued research we can tap the full potential of AI against natural disasters management. To prevent the horrendous aftermath of natural disasters, AI will one day become an invaluable tool.
The influence of Ai against natural disasters
The basis for understanding natural hazards is high-quality data. It is only once you’ve obtained it, that you can construct reliable AI algorithms. The emergence of novel data collection methods can help to gather high-quality data. Another useful approach would be exploring new ways to use traditional data collection methods.
One example is sensor networks which are handy for collecting data from topographically complex regions. These complex regions are tough to monitor at increased spatiotemporal resolutions. Such networks have facilitated the monitoring of flash floods and avalanches. Furthermore using snowpack and weather station data together can predict avalanche danger with human-level accuracy.
In Switzerland, an institute employs seismic sensors together with supervised machine-learning algorithms to detect tremors that come before avalanches. A lot of signals are recorded with seismic monitoring systems. However, avalanche signals have unique properties which permit AI algorithms to find them. Thus, eventually, you are left with accurate avalanche data. To predict the danger levels associated with avalanches simulation models can be created. In order to produce such models, data from weather stations in the Swiss Alps can be utilized.
The Focus Group on Artificial Intelligence for Natural Disaster Management is examining the possible ways of using AI against natural disasters. The group’s active topics include the use of AI for snow avalanches, floods, viruses, windstorms, etc.
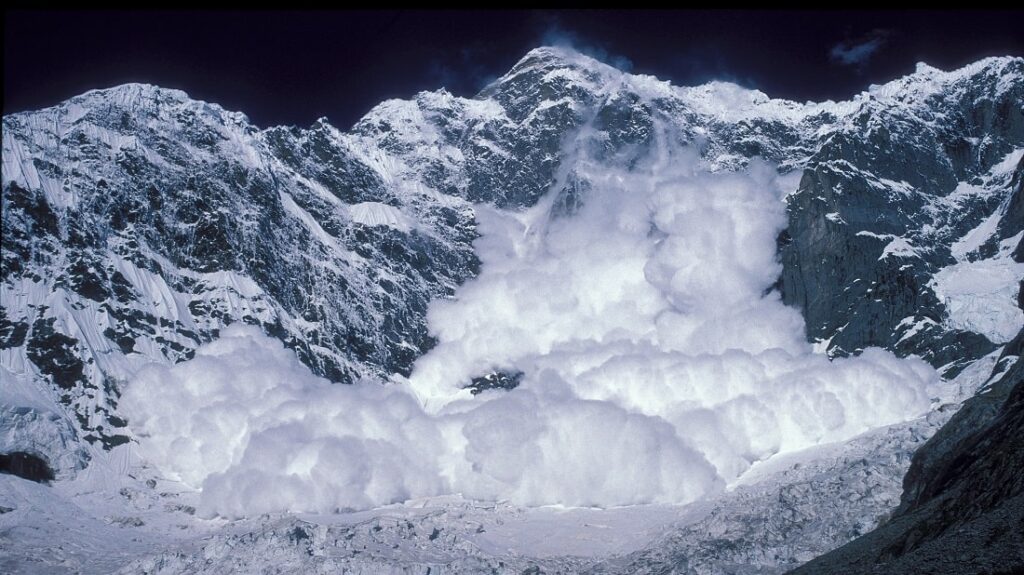
Flash Floods
Many countries have encountered horrible circumstances resulting from floods. ne example is Pakistan’s floods in 2022 that killed 1678 people. These floods washed away villages and destroyed their infrastructure. Over 3 million children were left at increased risk of waterborne diseases and malnutrition. In Puerto Rico, 900,00 people were left without power due to Hurricane Fiona. These horrendous circumstances could’ve been avoided had timely warnings been issued.
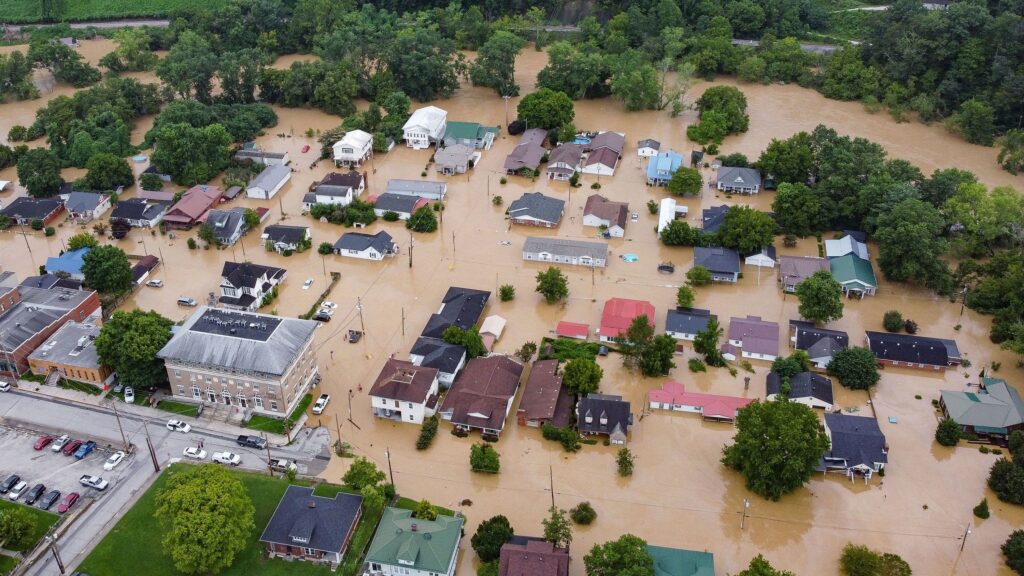
In Amsterdam, a team is trying to predict flood risks and storm surges by using deep learning methods. For this, they’ve analyzed various statistical models. They have used data from coastal areas all over the world. Today, with climate change and rising sea levels, it has become important to allow open access to data so that our increasingly vulnerable ecosystem can be saved.
Cities like Colima and Guadalajara are reverting to AI methods to detect floods. They are training ML models and comparing them with hydrological/ hydraulic models. After this comparison, they calculate performance metrics.
Tsunamis
Research centers around the world hold geological information that proves helpful in earthquake and tsunami detection. A reliable dataset is key to any prediction event. The monitoring of the Global Navigation Satellite System (GNSS) takes place at the Pacific Northwest Seismic Network. Traditional seismic systems prove inadequate in very large-magnitude earthquakes. Now such algorithms exist which estimate earthquake magnitudes within seconds of their occurrence. Tsunami amplitudes can also be predicted independently without the analysis of the triggering earthquake.
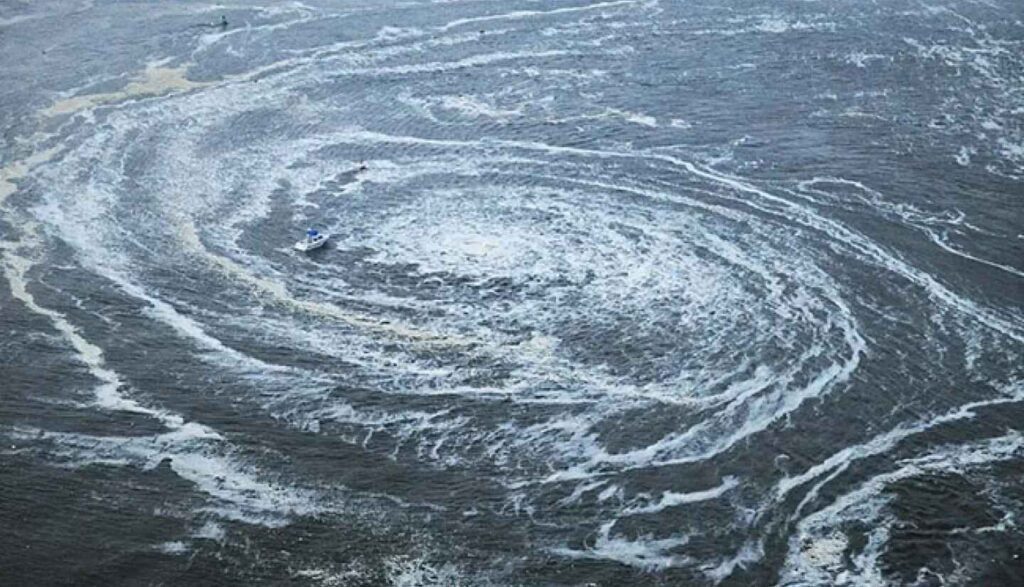
GNSS studies ground displacements and monitors any perturbations in ionospheric total electron content. However, some countries have limited bandwidth capacity. In these countries, AI can be used to transmit information that could potentially save lives. In developing countries, where the threat of tsunamis is high, AI methods for tsunami warning and damage assessment are crucial.
Hurricanes
The modeling of storms is making progress by leaps and bounds. Consequently, scientists are able to come up with better tools to deal with them. In 2013, when Cyclone Phailin hit Eastern India, the country was able to prevent the kind of disaster they faced in a similar storm 15 years ago. This was possible due to precise numerical modeling. This example highlights the use of AI against natural disasters.
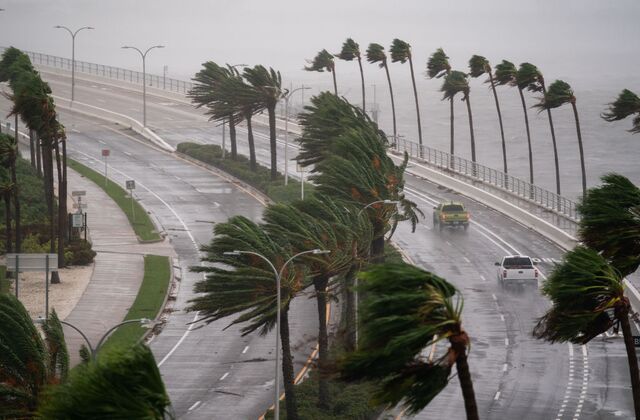
In fact, AI has the ability to determine the transition of a storm from a tropical storm to a hurricane and the other way around. This gives us better chances to prepare for hurricanes. As far as lightning storms are concerned, AI can conveniently predict their location, where they’ll hit, and which hotspots would catch fire. This gives us a chance to estimate the risk of a wildfire from a number of sources such as static electricity, friction, or hot surfaces. Many systems have been founded to protect people from natural hazards and need accurate forecasts and hazard maps for their operation.
Hazard maps are created using neural networks and can be made for severe convective events like hail and windstorms. Experts use an AI tool to predict the probability of seeing a convective event on a particular day at a specific place, given certain climate and meteorological settings. The ML model then concludes the days more prone to hail or windstorms. The AI tool uses older data to extrapolate to locations with data in short supply. In the end, these events are simulated with a numerical weather prediction model.
Limitations
For AI-based solutions, high-quality data sets are crucial. However, rare events offer only limited training data. To overcome this problem, multiple data sources or modeling approaches need to be combined. Unfortunately, even in the modeling phase, we have to encounter several limitations. For example, we don’t know for sure which modeling architecture is the best for our purpose or how to evaluate that algorithm. We need to design algorithms that are transferable to areas where data is limited. There is a lack of guidelines for researchers making these AI tools. Policymakers, governments, and humanitarian organizations also need a set of guiding principles to put the information supplied by these tools to service.
Future
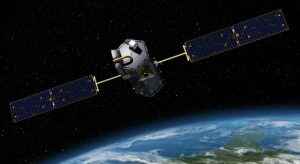
To address the problems stated above, we can take several steps in the future. New technologies such as the Internet of Things will allow the collection of more granular data. This will improve the quality of datasets. Quantum computing allows quicker and error-free calculations. Both academic and non-academic stakeholders should be encouraged to participate in scientific conferences. Research funding for such AI tools needs to be established. International standards should be set for the proper application of innovative disaster prediction technologies. Thus the future holds great promise for the use of AI against natural disasters.