There has been a growing trend of using AI for livestock management. The recent advances in artificial intelligence have led farmers towards an easier path of livestock maintenance. They can monitor their cattle and take action at the right time. This makes life easier for farmers, consumers, and even livestock animals.
AI in Livestock Feeding
Most farmers, from both small and large dairy farms, don’t understand the quality of fodder or its impact on milk production. However, now it’s possible for livestock management to use IoT devices to monitor animal feeding and drinking patterns. In order to achieve this, the algorithm employed in these devices is fed with an extensive dataset. Finding the best feeding pattern requires that the farmer test various configurations.
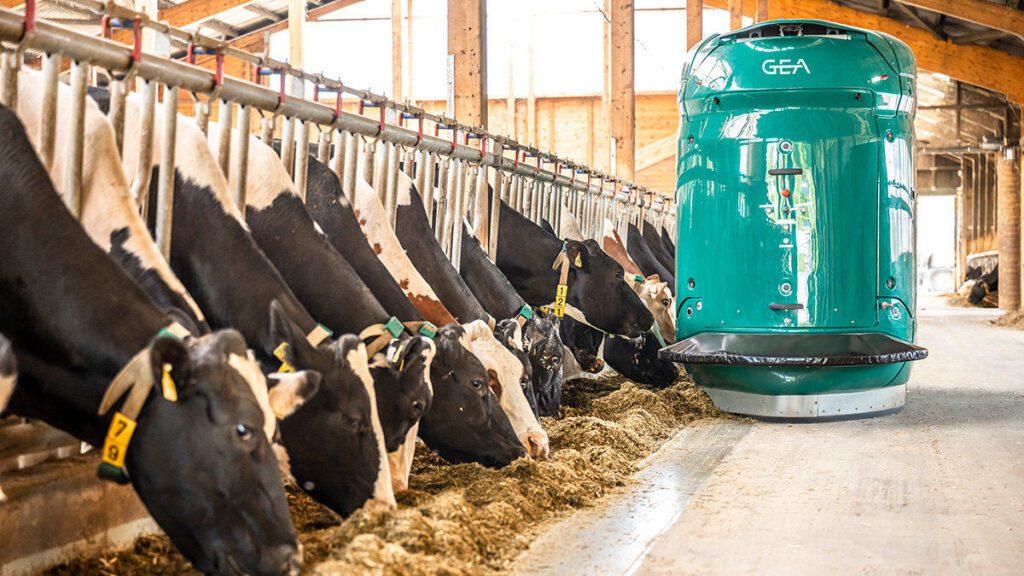
It is important for farmers to have a knowledge of the nutritional requirements of animals. They should also be aware of the nutritional makeup of different food sources. Equipped with this knowledge farmers have been successful in preventing the oversupply of nutrients at the herd and flock level. Livestock management is also embracing the concept of precision nutrition.
A study revealed that feeding pigs according to customized diet plans reduced their lysine intake by 26 %. It also lessened their nitrogen excretion by 30%. This reduction took place without compromising the pigs’ performance in any way. There is a program that allows electronic feeders to feed individual sows according to their properties such as weight, age, parity, and gestational stage. Such feeding has advantages such as waste reduction and increased lactation.
AI produces a digital twin or avatar which creates a digital replica of each animal by using the information about feed mills or feeding systems. These twins are used to test different scenarios at a low cost. For example, you can find out the possible nutritional composition of shipped foods. You can also use this information to construct models for testing the contamination situation. For instance, what conditions favor a particular bacteria or mold’s survival and how those conditions can be altered.
AI Powered Disease Monitoring for Livestock
Using AI, it becomes possible to spot conditions such as lameness in cattle. AI also alerts farmers when piglets or chickens experience stress. Interestingly, new technology allows the detection of parasite or insect proliferation. One method of pathology detection is the analysis of feces. The AI system can then immediately identify the potential danger of contamination. This mechanism is an integral part of epidemic prevention practices. Thus computer vision automates this inspection.
Farm animals often have to endure heat because they are kept together in a limited space. AI-powered sensors not only monitor the heat stress faced by animals but also link it to particular behaviors or events. These machine-learning models are able to issue alerts as soon as the temperature crosses a safe level. The data gathered is used to further polish this program.
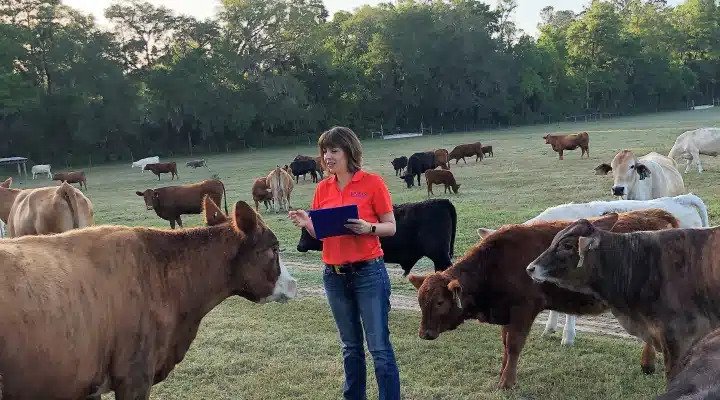
In the area of disease detection, we also use livestock vocalizations. The extraction of audio data from animal recordings is followed by training machine learning algorithms. After that, anomalies in vocalization are recognized and classified. All these different information sources are combined to give farmers a complete picture of the state of their animals.
Reproduction and Breeding
Livestock breeding requires the proper designing of novel mating plans. AI allows uninterrupted farm monitoring and is used for livestock management even in the absence of a breeder. Thus breeders can use their valuable time to complete tasks of more significance. Cameras strategically placed in livestock shelters provide the data. This is submitted to neural networks that detect situations and alert farmers, veterinarians, and technicians. These people then decide which course to take.
Innovative mating plans use biotechnology as well as statistical models. Today some open-source tools, such as XSimV2 are present which allow simulation of breeding programs. The two major breeding steps are mate and select. It is possible to assemble these functions in many random combinations. This tool will then present as many designs as possible to facilitate users. It also has the latest information on reference genomes of breeding animals, such as cattle and pigs.
Genetics and AI for Livestock”
Advanced statistics is the heart of artificial intelligence and also forms the base of animal breeding and genetics. That is why people combine the two. This gives an opportunity to use AI for livestock management. Furthermore, the core requirement of designing mating plans is making predictions. Artificial Intelligence for Livestock makes this too convenient. Data mining techniques are quick, cheap, and have high accuracy. They are capable of dealing with complex, imprecise, and noisy data. Handling such data is impossible using conventional techniques.
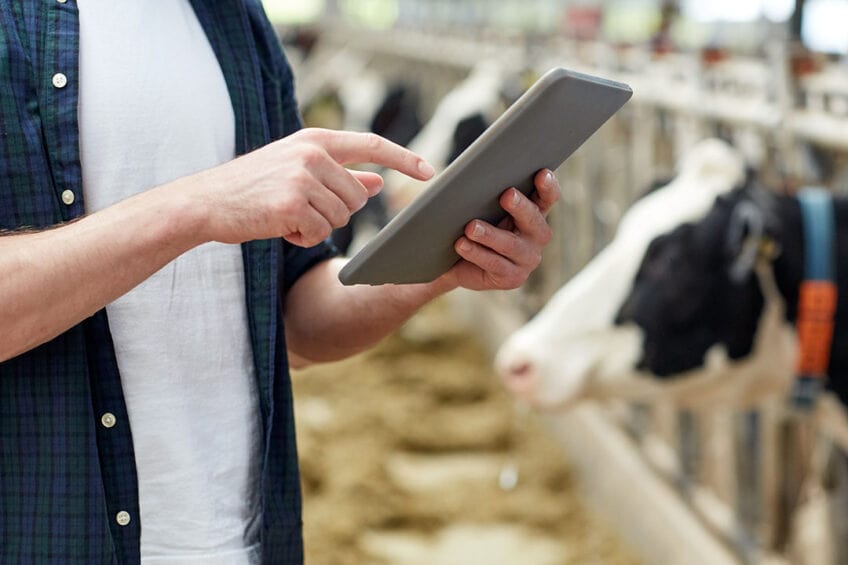
Machine learning is useful in predicting genomes. When genetic architecture is complicated, this becomes especially true. ML can recognize SNPs and link them to candidate genes. Genome-wide association studies link genes to diseases. Machine learning facilitates such studies. A process called imputation predicts missing marker genotypes. Studies have demonstrated that ML methods when used for imputation give higher accuracy. ML models filter and edit data and provide better phenotype quality checks. Farm managers can detect animal body weight from just body images instead of using a weight scale.
Carcass Evaluation
Carcass grading assesses the quality of culled cows and bulls. The US Department of Agriculture defines these grades. Automated meat grading systems simulate the reasoning skills of real-life human meat graders. This presents another area for the use of AI for livestock management. They use a computer vision system and consist of a subsystem that acquires the carcass characteristics. the knowledge-based meat grading subsystem uses this information and sets rules for finalizing the grade results.
AI-driven automated systems collect data about the amount of fat present on a subprimal. They also check other dimensions such as weight. They are capable of identifying contamination. This data can even be sent back to animal production units that monitor animal genetics, nutrition. and growth. This further optimizes production programs. Further down the road, these systems examine package integrity and verify that the correct product was shipped to the target consumer. AI avoids human errors and hence improved quality leads to improved profitability. AI-powered automation `brings the possibility of a single robot performing multiple tasks. The different cutting tools allow users to make cuts at required orientations and can even allow multiple cuts in unison.